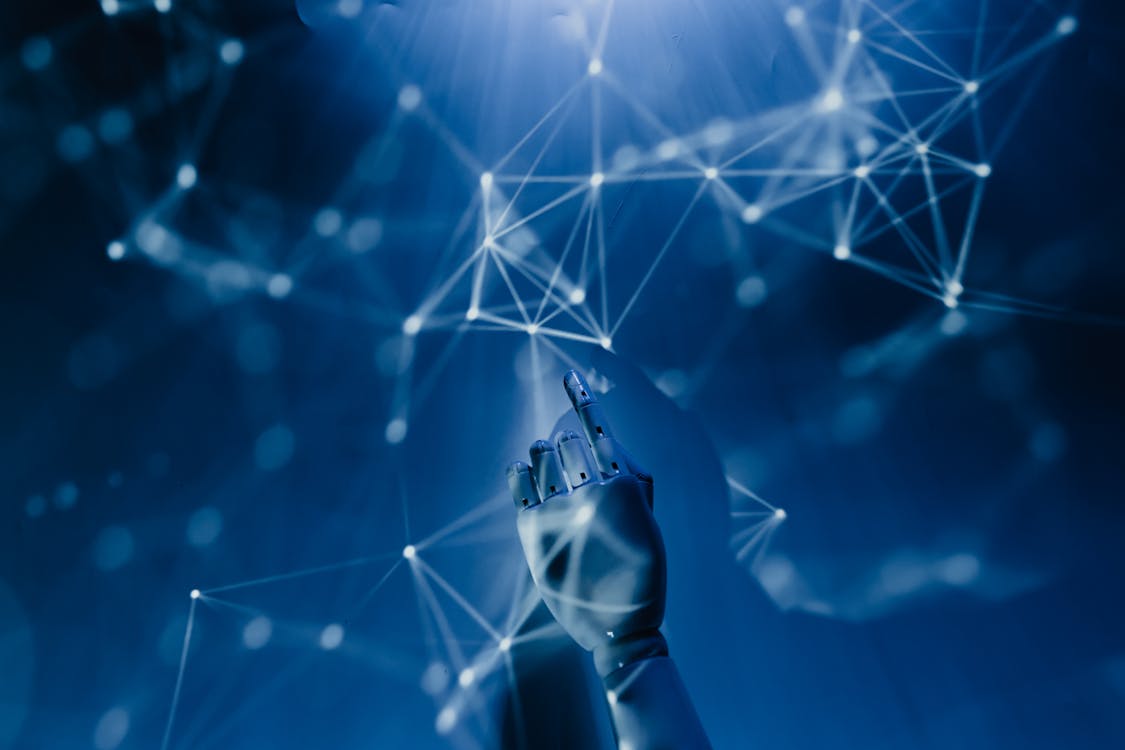
Are you ready to revolutionize your investment strategy? In today's fast-paced financial world, making data-driven decisions with AI is no longer optional—it's essential. Whether you're a seasoned investor or just starting out, this comprehensive guide will show you how to harness the power of AI to make smarter, more informed investment choices. We'll explore the exciting intersection of data science and finance, revealing how you can stay ahead of the curve and potentially boost your returns. Let's dive into the world of AI-driven investing and discover the secrets to making data-driven decisions that could transform your portfolio.
Introduction
The investment landscape has undergone a dramatic transformation in recent years. Gone are the days when gut feelings and hunches ruled supreme. Today, the most successful investors are those who embrace data-driven decision-making, leveraging the power of artificial intelligence to gain a competitive edge.
Making data-driven decisions in modern investing isn't just a trend—it's a necessity. With the sheer volume of information available, human analysts alone can no longer process and interpret all the relevant data quickly enough to stay ahead of the market. This is where AI comes in, offering the ability to analyze vast amounts of data in real-time, identify patterns, and generate insights that would be impossible for humans to discern on their own.
Understanding Data-Driven Decisions in Investing
At its core, making data-driven decisions in investing means basing your investment choices on objective data and statistical analysis rather than subjective opinions or emotions. This approach relies on collecting and analyzing large datasets to identify trends, patterns, and correlations that can inform investment strategies.
Compared to traditional investment approaches, which often rely heavily on intuition and experience, data-driven investing offers several advantages. It can help reduce bias, improve consistency, and potentially lead to better outcomes over time. However, it's important to note that data-driven investing isn't about completely removing human judgment from the equation—rather, it's about augmenting human expertise with powerful analytical tools.
The Role of AI in Making Data-Driven Investment Decisions
Artificial Intelligence is revolutionizing the way we make data-driven decisions in investing. AI algorithms can process and analyze vast amounts of data much faster than humans, identifying patterns and trends that might otherwise go unnoticed. This capability allows investors to make more informed decisions based on a broader range of information.
In financial analysis, AI is being applied in numerous ways, with Large Language Models (LLMs) playing an increasingly significant role. For example, LLMs can be used to analyze vast amounts of financial reports, SEC filings, and earnings call transcripts, extracting key insights and trends that human analysts might miss. These models can also assist in generating comprehensive investment memos, summarizing complex financial data, and providing context-aware responses to investor queries. Additionally, AI-powered tools can help in portfolio optimization by processing and interpreting large datasets of market information, economic indicators, and company fundamentals. Computer vision technologies are also being employed to analyze alternative data sources, such as satellite imagery of industrial facilities or shipping ports, to gain unique insights into economic activities and supply chain dynamics.
Key Components of Making Data-Driven Decisions with AI
Effective data-driven decision-making in investing relies on several key components. First and foremost is data collection and management. This involves not only gathering relevant financial data but also ensuring its quality, consistency, and accessibility.
Equally important are the tools and techniques used to analyze this data. These can range from simple statistical methods to complex machine learning algorithms. For instance, regression analysis might be used to identify relationships between different economic variables, while clustering algorithms could be employed to group similar stocks or market conditions.
Implementing Data-Driven Decision-Making in Your Investment Process
Transitioning to a data-driven investment approach is a gradual process that begins with assessing your current investment strategy and identifying areas where data analytics and AI could add value. This might involve automating routine tasks, enhancing risk assessment, or improving market analysis.
Once you've identified opportunities for AI integration, the next step is choosing suitable tools and platforms. The market now offers a range of AI-powered investment solutions, from robo-advisors for individual investors to sophisticated analytics suites for institutional use. For instance, Junrs has emerged as a valuable platform in this space, combining AI-driven insights with traditional financial metrics to streamline the investment analysis process and support data-driven decision-making.
Selecting the right tools is crucial, but equally important is training your team to effectively use these AI-assisted decision-making systems. While not everyone needs to become a data scientist, team members should understand the basics of data analysis and how to interpret AI-generated insights. This knowledge empowers them to leverage platforms like Junrs more effectively, blending AI-powered analysis with human expertise to make more informed investment decisions.
By integrating such tools and fostering a data-driven culture, you can significantly enhance your investment process, making it more objective, efficient, and potentially more profitable in the long run.
Challenges and Considerations in Making Data-Driven Decisions
While data-driven decision-making offers many benefits, it's not without challenges. One of the most significant is ensuring data quality and reliability. In the world of investing, where decisions can have major financial implications, working with accurate and timely data is crucial.
Ethical considerations also come into play when using AI in investing. For example, how do we ensure that AI algorithms don't perpetuate or exacerbate existing biases in financial markets? There's also the question of transparency—how can we understand and explain the decisions made by complex AI systems?
Finally, it's important to strike the right balance between AI insights and human judgment. While AI can process vast amounts of data and identify patterns, human expertise is still crucial for interpreting these insights in the context of broader market dynamics and real-world events.
Case Studies: Successful Implementation of Data-Driven Decisions with AI
To illustrate the power of data-driven decision-making in investing, let's look at a couple of real-world examples.
Consider Bridgewater Associates, one of the world's largest hedge funds. They've long been at the forefront of using data and technology in investing. Their approach involves using AI to analyze vast amounts of economic and market data, helping them to identify trends and make investment decisions. While the specifics of their strategies are closely guarded, their success—managing over $140 billion in assets as of 2021—speaks to the potential of data-driven investing.
On a smaller scale, robo-advisors like Wealthfront and Betterment have made data-driven investing accessible to individual investors. These platforms use AI algorithms to create and manage diversified portfolios based on each investor's goals and risk tolerance. By automating many aspects of the investment process, they're able to offer sophisticated investment strategies at a fraction of the cost of traditional financial advisors.
Future Trends in Data-Driven and AI-Powered Investment Decisions
The field of AI-powered investing is evolving rapidly. One emerging trend is the use of alternative data—information from non-traditional sources like satellite imagery, social media, or IoT devices—to gain unique insights. For example, some hedge funds are using satellite images of parking lots to estimate retail sales before official figures are released.
Another exciting development is the application of reinforcement learning in trading algorithms. These AI systems can learn and adapt their strategies in real-time based on market conditions, potentially leading to more robust and flexible investment strategies.
As AI becomes more integral to the investment process, the role of data scientists in finance is evolving. Instead of simply building models, they're increasingly involved in strategic decision-making, working closely with portfolio managers to develop and implement AI-driven investment strategies.
Conclusion
Making data-driven decisions with AI is transforming the world of investing. By harnessing the power of data analytics and machine learning, investors can gain deeper insights, manage risk more effectively, and potentially achieve better returns.
However, it's important to remember that AI is a tool, not a magic solution. Successful investing still requires human expertise to interpret AI-generated insights, make strategic decisions, and navigate the complexities of financial markets.
As we look to the future, one thing is clear: the investors who thrive will be those who can effectively combine the analytical power of AI with human judgment and expertise. By embracing data-driven decision-making and staying abreast of AI advancements, you can position yourself at the forefront of this exciting new era in investing.